Introduction
The idea of machines helping doctors has been around for a while but with the rise of generative AI, that idea is no longer science fiction. Today, AI is stepping into roles that involve creating rather than just analysing. It’s writing medical reports, simulating molecules for new drugs, and even helping radiologists spot hard to see patterns.
Generative AI is becoming an invisible partner in healthcare, quietly reshaping everything from how drugs are developed to how diseases are diagnosed. And the best part? It’s just getting started.
Key Components
To understand how generative AI is helping healthcare, we first need to look at the key building blocks:
- Massive datasets: Generative AI thrives on examples—medical records, lab results, X-rays, molecular structures. The more diverse and accurate the data, the better the output.
- Model training: These AI systems “learn” from past cases, absorbing patterns in disease, drug interactions, and even patient behaviour.
- Generation layer: Instead of just recognizing a tumour or flagging an abnormality, generative AI can write a summary, simulate a treatment plan, or create entirely new possibilities like a drug molecule that’s never existed before.
It’s not replacing doctors or researchers. It’s acting like a turbocharged assistant faster, tireless, and great with detail.
Types of Generative AI in Healthcare
Generative AI takes many forms in the medical world, each tailored to specific needs:
- Text-based generation: Think AI writing discharge summaries, clinical notes, or patient-friendly explanations of medical conditions. Tools like ChatGPT are being adapted for medical writing to save doctors time and reduce burnout.
- Image generation and enhancement: AI models can generate high-quality synthetic medical images (like MRIs or CT scans) to train other systems or fill in gaps in patient data.
- Molecular generation: Perhaps the most groundbreaking AI can “imagine” new drug molecules that might bind to a target protein and stop disease at the source.
- Predictive models: These use patient data to forecast future health risks or outcomes, helping doctors make more informed decisions.
Each type plays a role at different stages—before diagnosis, during treatment, or even long after recovery.
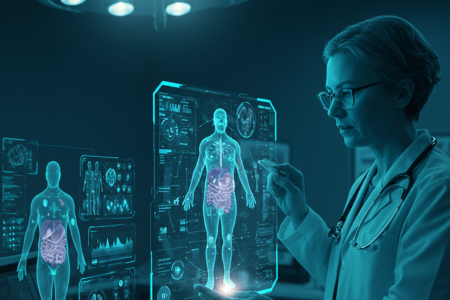
Key Benefits and Challenges
Benefits
- Speed: Discovering a drug used to take over a decade. Now, AI can suggest viable molecules in weeks.
- Personalization: AI can analyse a person’s entire health history and tailor treatment plans specifically for them.
- Efficiency: By generating paperwork, reports, and routine summaries, AI gives healthcare workers more time to focus on patients.
Challenges
- Trust: Would you be comfortable knowing your treatment plan was influenced by an AI? Many patients (and doctors) still aren’t sure.
- Bias in data: If the AI is trained mostly on data from certain populations, it may miss signs in underrepresented groups.
- Regulation: Healthcare is heavily regulated for good reason. Getting AI systems approved for clinical use can take years, even if they work well.
The promise is huge, but the path forward must be careful and ethical.
Real-Time Applications
Generative AI isn’t just an idea it’s already working behind the scenes:
- Drug discovery: Startups like Insilico Medicine and big players like Google DeepMind are using generative models to invent molecules that could treat cancer, rare diseases, and infections.
- Radiology reports: AI can scan an MRI and generate a full report in seconds, which radiologists can review and approve. This saves time and cuts backlogs.
- Chatbots for triage: Some clinics use AI-powered bots to ask patients questions, narrowing down potential causes before they even see a nurse.
- Synthesizing health data: AI can create synthetic patient records to test new hospital software without using real patient data.
We’re seeing these tools not just in research labs, but in hospitals, clinics, and telehealth apps.
How It Works
Let’s say a researcher wants to find a drug that treats a certain cancer. Traditional research might involve testing thousands of molecules over years. With generative AI, the model can simulate what a working molecule could look like based on everything it has learned from past chemical structures and successful drugs.
Or imagine a radiologist looking at a scan. AI can be trained to generate a report, flag unusual areas, and even compare it with similar past scans to help with diagnosis.
It’s like having a second set of eyes ones that have seen millions of cases and can spot things a human might miss.
Getting Started with Generative AI in Healthcare
You don’t have to be a researcher to explore the potential of AI in medicine. Here’s how anyone from students to professionals can begin:
- Learn the basics: Platforms like Coursera, edX, or even YouTube have beginner-friendly courses on AI in healthcare.
- Explore tools: Try GPT-based medical note generators or explore AI-powered research assistants like SciSpace or Elicit.
- Stay informed: Follow companies like DeepMind, PathAI, or BioNTech to see how AI is being applied in real-world settings.
- Join the conversation: Whether you’re a medical student, doctor, or just interested in tech, there are growing communities (like AI Med or LinkedIn groups) where these developments are openly discussed.
Curiosity is the first step understanding how it fits into your world comes next.
Conclusion
Generative AI in healthcare is more than a buzzword it’s becoming a critical tool in how we treat, diagnose, and care for people. It won’t replace doctors, but it can empower them to make better decisions, faster and with more information.
Whether it’s helping discover the next life-saving drug or easing the workload on frontline nurses, generative AI is opening doors we didn’t know existed just a few years ago.
The future of medicine won’t be written only in labs or hospitals it will also be generated, one breakthrough at a time.