Introduction
If you’ve ever used an app that turns your selfie into a painting, created fantasy landscapes from a single sentence, or scrolled through AI-generated art online and thought, “How does this even work?” you’ve likely seen the results of GANs and Diffusion Models, the two major forces behind AI-generated imagery today.
Though both are utilized to develop incredible visual material, they do so in fairly dissimilar methods. In this weblog, we’re going to see what exactly these models are, how they compare, and how they are making the art and creativity future brighter. No need for any technical background all it takes is some curiosity.
Key Components
At the heart of AI-created artwork are systems that learn from vast libraries of genuine images hundreds of millions of pictures, sketches, and paintings to see how visuals tend to be constructed. They don’t steal pictures, but learn the style, colour scheme, and textures to create something fresh.
Both Diffusion Models and GANs (Generative Adversarial Networks) are based on this concept of learning from examples. What they want to do is create images that have a realistic, artistic, or surreal feeling depending on how the user wishes. Although they have varying approaches, both seek to provide AI with the capability to create and not merely analyse.
Types of AI Art Models: GANs vs. Diffusion
GANs: The Artists That Learn by Competition
GANs function in the manner of a game of two players: one generates deceptive images, while the other is the judge determining if they’re real or false. As time passes, the creator becomes skilled at deceiving the judge. This give-and-take refines the images to become more detailed and believable. GANs were one of the pioneering models to disrupt the AI artwork, particularly those tools that create photorealistic faces or abstract images.
Diffusion Models: The Slow and Steady Painters
Diffusion models are more gradual, step-by-step in their approach. Rather than generating an image directly, they begin with raw noise (imagine static on a TV screen) and gradually “clean it up” step by step until a distinct image emerges. It’s like painting in reverse beginning with a messy canvas and gradually bringing out the masterpiece beneath. Such models, such as those behind DALL·E 2, Stable Diffusion, or Midjourney, have become the standard for today’s text-to-image generators.
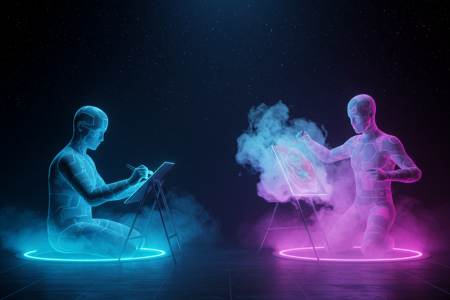
Key Advantages and Drawbacks
Both categories of models possess advantages.
- GANs are quick and excellent at creating sharp, high-resolution images, particularly when trained on objects such as faces or clothing. But they can be lacking at times in terms of consistency or imagination, particularly on intricate or imaginative sets.
- Diffusion models, meanwhile, are very versatile. Present them with an odd or surreal prompt such as “a cat surfing on Saturn at sunset” and they’ll have a go at it. They’re usually slower and need more computational power to produce output, though.
- Both are causing concern about copyright, authenticity, and the morality of producing art that could be perceived as having originated from a human artist, particularly when trainedon actual artists’ work without their consent.
Real-Time Applications
AI-generated art is entirely more than a specialist or pastime activity it’s appearing in day-to-day tools and sectors.
- Marketing & Advertising teams utilize AI-generated pictures to ideate visuals, make mock-ups, or rapidly try out design concepts.
- Concept art and world-building are done by game developers and movie studios using these tools.
- Social media content creators and influencers use AI to create profile images, backgrounds, and stylized graphics.
- Print-on-demand companies utilize AI to create one-of-a-kind art for posters, t-shirts, and merchandise.
- Even casual users toy with AI art in apps such as Lensa, WOMBO Dream, or Canva’s AI design capabilities.
The distinction between AI-augmented creativity and human-created art is rapidly disappearing.
How It Works
With GANs, it’s similar to teaching a child to draw by letting them draw pictures and having another child critique them. Over time, the first child knows what’s good and what’s not. This “duel” enhances the quality of every sketch so that eventually the drawings are incredibly lifelike.
With Diffusion Models, it’s not so much like shining a light on the image. It’s like cleaning a foggy mirror. The image is there just wait until it slowly and gently reveals itself. The model works with a cloud of noise and gradually step-by-step retrieves the shapes, colours, and details that make up the final image.
Both techniques are dependent on hundreds of examples and plenty of trial and error to improve. And both require high-performance computers to function efficiently, particularly for high-quality or high-resolution images.
Conclusion
Whether it’s GANs vying to generate the ultimate image or diffusion models gradually crafting something from digital mess, these two forms of AI are at the centre of the new art revolution. They’re not replacing artists they’re presenting new means to envision, design, and co-create with technology.
With generative AI getting easier to use, anyone artist or not can bring an idea to life visually, quickly. The software already belongs in your pocket. The only question remaining is: what will you make?